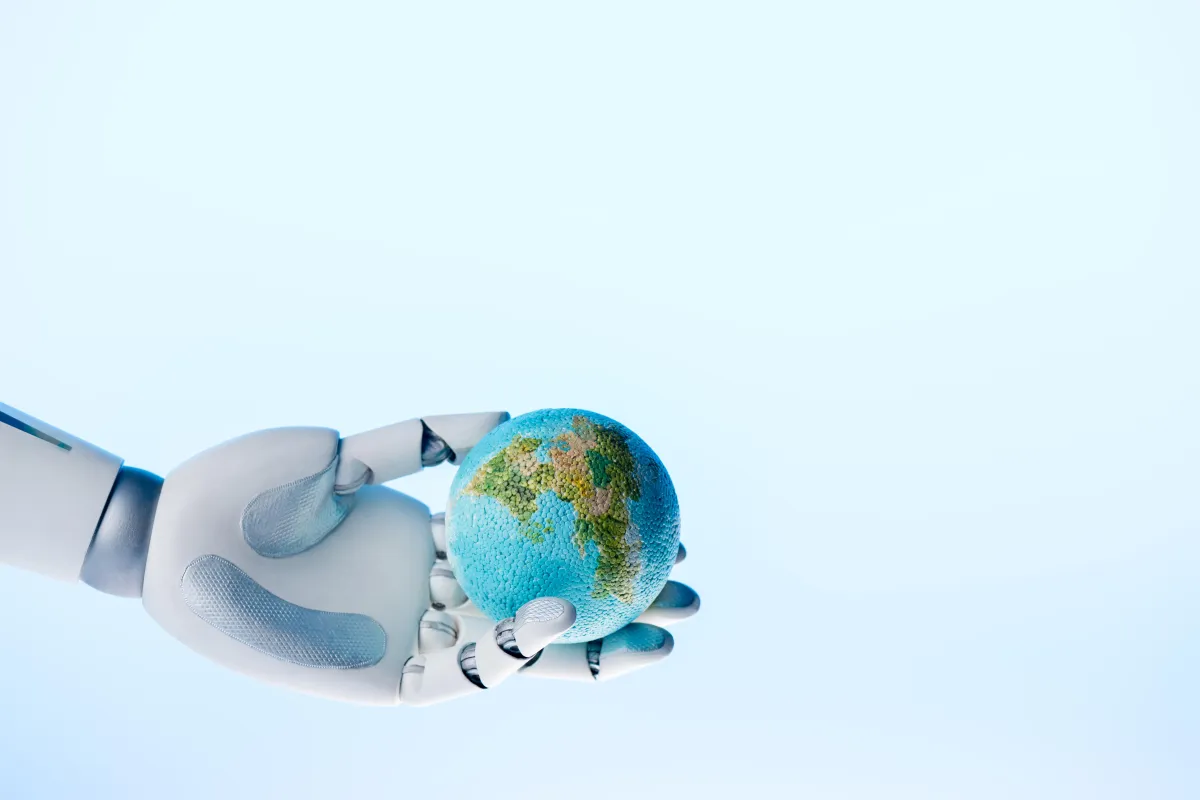
Why Generative AI is Bad for the Climate
Why Generative AI is Bad for the Climate
As artificial intelligence (AI) continues to evolve, generative AI has emerged as one of the most revolutionary technologies of the last decade. Capable of creating text, images, music, and even entire virtual environments, generative AI offers unprecedented potential in industries ranging from entertainment to education. But as with many technological advancements, generative AI comes with a cost—specifically, a significant environmental one.
While AI's positive contributions are undeniable, it's becoming increasingly clear that the energy demands of training and operating large AI models are contributing to the climate crisis. In this blog, we’ll dive into why generative AI is bad for the climate, the scope of its carbon footprint, and what can be done to mitigate its environmental impact.
1. The Energy-Intensive Nature of Generative AI
Generative AI models, like OpenAI’s GPT series or Google’s DeepMind, are built on deep learning, a branch of machine learning that requires vast computational resources. These models are trained using enormous datasets that take weeks or even months of continuous computing to process. This training is done on powerful servers that consume immense amounts of energy.
Key Factors Contributing to AI’s Energy Use:
Massive Data Processing: Training a large AI model involves processing massive amounts of data to "teach" the AI how to generate new content. This requires enormous computing power.
GPU-Intensive Computing: The servers used to train these models rely heavily on high-performance GPUs (graphics processing units) and TPUs (tensor processing units), which consume significant energy over time.
Cloud Infrastructure: Most AI models are trained in data centers that run 24/7. These data centers require electricity to power the servers and cooling systems to prevent overheating, adding to their overall energy consumption.
While AI brings incredible breakthroughs in automation, creativity, and problem-solving, the sheer energy required to power generative AI models is leading to an increasingly unsustainable environmental footprint.
2. The Carbon Footprint of AI Training
To understand the environmental impact of generative AI, it’s essential to consider the carbon footprint created by training large AI models. Researchers have found that training a single large AI model can emit as much carbon as the lifetime emissions of five cars, including manufacturing and fuel consumption.
Case Study:
A 2019 study estimated that training a transformer-based AI model (like GPT-3) can result in CO2 emissions exceeding 284 metric tons. To put that into perspective, the average person generates around 4 metric tons of CO2 per year.
This figure doesn’t account for the multiple versions of the model that are trained during research and development. AI developers often train several models, testing different configurations before releasing the final version. Each of these iterations adds to the overall carbon emissions.
While the AI industry pushes forward with increasingly complex models, these systems' environmental costs must be acknowledged and addressed.
3. The Impact of Data Centers on the Environment
Data centers, where most AI models are trained, are a major contributor to the environmental costs of generative AI. As AI becomes more mainstream, the demand for cloud computing services provided by tech giants like Amazon, Google, and Microsoft has skyrocketed. These data centers house the powerful servers necessary for AI training, but they also consume vast amounts of energy.
Environmental Issues Associated with Data Centers:
High Energy Demand: Data centers require a continuous power supply to run the servers that train AI models. The demand for energy is so significant that data centers account for 1% of global electricity consumption—a figure that continues to grow.
Cooling Requirements: Servers generate enormous amounts of heat, and keeping them cool is vital to prevent overheating and equipment failure. Cooling systems are highly energy-intensive and contribute to the overall environmental impact.
Fossil Fuel Dependency: While many companies claim to be moving towards renewable energy sources, a large portion of data centers worldwide are still powered by electricity derived from fossil fuels. This reliance on non-renewable energy further exacerbates the carbon footprint of AI operations.
While there are efforts to transition data centers to green energy, the pace at which AI is advancing makes it difficult to offset the environmental damage.
4. The Hidden Energy Cost of AI Deployment
In addition to the energy-intensive nature of training AI models, running them in real-world applications also has an environmental cost. Once generative AI models are trained, they continue to consume energy each time they are used. For instance, when an AI model generates text, creates images, or engages in interactive tasks, it requires computational resources from cloud infrastructure or servers.
AI Usage and Its Continued Energy Demands:
Consumer Applications: As generative AI becomes integrated into mainstream apps (such as voice assistants, automated writing tools, or recommendation engines), the number of requests to servers grows exponentially, leading to ongoing energy consumption.
Business and Enterprise Use: Many industries are incorporating AI into their daily operations, from automating customer service to managing supply chains. As AI-powered applications scale up, so does the energy required to keep them running smoothly.
AI as a Service (AIaaS): Companies offering AI as a cloud-based service (such as Microsoft Azure, Amazon Web Services, and Google Cloud) are rapidly expanding their AI capabilities. However, offering AI tools on-demand also means more data centers, more energy, and more environmental impact.
5. How AI Developers Are Trying to Reduce the Environmental Impact
While generative AI undoubtedly has a significant environmental cost, there are efforts within the AI community to reduce the technology’s carbon footprint. Several initiatives are being explored to balance the growing demand for AI with environmental sustainability.
Green AI Initiatives:
Efficient Algorithms: Researchers are working on developing more energy-efficient algorithms that require less computational power to train AI models. Optimizing code and reducing unnecessary computations can lead to a reduction in energy use.
Renewable Energy Sources: Tech companies like Google, Microsoft, and Amazon have committed to running their data centers on 100% renewable energy. While this is a positive step, the transition to green energy is a slow process that still leaves gaps in reducing AI’s carbon footprint.
AI Model Compression: Model compression techniques are being developed to reduce the size of AI models without sacrificing performance. Smaller models require less energy to train and operate, making them more eco-friendly.
Carbon Offsetting: Some companies are purchasing carbon offsets to neutralize the emissions generated by AI operations. While carbon offsetting can help reduce net emissions, it doesn’t solve the underlying problem of high energy consumption.
Despite these efforts, the rapid growth of generative AI raises questions about the long-term sustainability of the technology.
6. Balancing AI Innovation with Environmental Responsibility
While generative AI offers immense potential for industries across the board, its environmental cost cannot be ignored. The technology’s reliance on energy-intensive data centers and computational power highlights the need for a balanced approach—one that prioritizes both innovation and environmental responsibility.
Ways to Balance Innovation with Sustainability:
Promote Research into Green AI: More investment is needed to develop energy-efficient AI algorithms and systems. Governments, academic institutions, and tech companies should collaborate to fund research focused on reducing the environmental impact of AI.
Incentivize Renewable Energy Use: Governments and international bodies can incentivize tech companies to adopt renewable energy sources for their data centers. Tax breaks, subsidies, and regulatory support can help accelerate the transition to green energy.
Develop Industry Standards: Creating industry standards around energy consumption and carbon emissions for AI development could push companies to adopt more sustainable practices. Standards would ensure that companies prioritize energy efficiency in their operations.
Encourage Responsible AI Use: Companies and consumers should be aware of the environmental impact of AI and strive to use it responsibly. This might involve limiting unnecessary AI use or opting for more efficient models when available.
Generative AI is a remarkable technological advancement, but it comes with a hidden environmental cost. From the energy required to train AI models to the ongoing power demands of data centers, the carbon footprint of AI is significant. To fully harness the benefits of AI without exacerbating the climate crisis, the tech industry must continue developing greener, more sustainable AI solutions.
Has your business been impacted by a natural disaster or major business disturbance?
Click the “Get Assistance” button to begin the process—we are here to help!